From the Burner Phone

“HIMSS hasn’t announced attendance because it is down thousands over last year. It would have been even worse except for the 10 percent of the attendees who were international.” I was expecting to see attendance figures released today, along with all the other standard announcements that go out on the same conference day every year. Traffic seemed markedly down in the exhibit hall and elsewhere. I will lose a lot of respect for HIMSS if they can’t put their ego in check and announce the conference stats as usual regardless of whether they are flattering, especially when they’ve been pushing “45,000+” in our faces for weeks.
“Few CEOs were in the exhibit hall. Lots of dead booths and vendors complaining about reduced traffic.”
“We’re firing KLAS this week. Too little value for way too much money.”
“Quoting an ‘EHR extraction’ expert walking around at HIMSS. CMS is basically saying the Trump administration is wanting to prove that the Obama administration was sloppy with giving out all this MU money without any oversight. They are going after the big vendors to recover it. This is just the first of many.”
“It’s interesting how many executives were sitting in hotel lobbies Tuesday morning with their roller bags, obviously not planning to stick around for anything beyond the pre-conference team meeting and the first day’s booth opening. I bet that left some nice suites vacant from Tuesday night on.“
“Do all those social media ambassadors realize that their phones can be set to take photos toward the front instead of just facing themselves?”
Observations
Today started out surprisingly chilly and rainy, with people who were waiting on the shuttle buses forced to seek shelter or create impromptu head coverings from jackets or plastic grocery store bags. Many of them called Lyft, I noticed, also observing that I didn’t see any Uber riders even though I thought years ago that Uber would swat Lyft like a bug. Traffic was slow and my bus took 45 minutes to travel a couple of miles. My bus arrived at the convention center at nearly 10:00, fidgeting like smokers on a long flight as they contemplated the bleak picture of their exhibit hall booths open without them. They were not only late, but irrecoverably mussed from the rainy, windy start of the day.
The shuttle bus had a QR code on the wall, so of course I had to scan it. It brought up a YouTube safety video, which if you are sinking in a Florida drainage pond or feeling gasoline-fueled flames licking your legs, might not be the most productive use of your time.
I forgot to mention that HIMSS gave exhibit hall vendors orange badge lanyards this week vs. the black ones the rest of us have. That’s a step up for exhibit hall staff, though, since before this year, HIMSS gave nothing except the lowest-rent form of clip-on throwaway plastic badge holder like you would buy in flimsy boxes at Office Deport. The two-lanyard system recalls the old days of HIMSS, when the badges of vendors and providers were dot-matrix printed on different colored paper (yellow and blue, as I recall).
I mentioned yesterday that I decided that it doesn’t make sense for me to to spend a large fortune for a small, remotely located booth that draws few visitors, so I’ll pass on exhibiting at HIMSS20 for the first time in a few years. Our booth was quite busy today as a result, with readers paying their respects to the retiring Smokin’ Doc. It was actually kind of moving, like watching people honoring a retiring colleague.

It was a big readership day today, with 9,000 page views. Thanks to every HIStalk reader, whether you keep coming back because you love it or because you hate it.
Tomorrow is exhibitor bug-out day, as it is not only the last (and shortened) exhibit hall day, but also Valentine’s Day. I guarantee that checkout luggage will fill booths in the morning and the hall will start emptying out by noon, as several vendor employees told me they’ve booked early flights to get home to their loved one. HIMSS policy has an unenforced requirement to stick around until the bitter end (which we never do) but nobody really cares, especially this year, when exhibitors may already be cranky about attendance and traffic. Walk the hall around lunchtime and all that good swag that vendors were protectively hiding will be freely available because they won’t want to pack it up and take it home.
AMA clarifies that the bar I saw set up outside their booth Tuesday afternoon wasn’t a happy hour, but rather the setup for an invitation-only event reception for women leaders in business. I wasn’t judging, but that’s good to know.
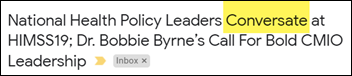
This is definitely some odd wording, especially from an industry magazine’s email blast, although the whole thing had a lot of awkward prose and punctuation like maybe the author learned English later in life. A couple of readers sent this my way.
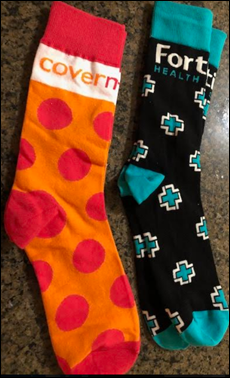
I decided to wear my new socks from Fortified Health Security today, then the CoverMyMeds ones tomorrow.
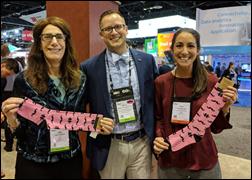
Nathan Grunewald, MD gave Epic’s Judy Faulkner a pair of pink socks and got her to pose for a photo besides. Note also that unlike most company CEOs who aren’t billionaires, Judy was working the Epic booth. I admit that despite trying to figure it out, I have no idea why pink socks have anything to do with healthcare or why people wearing them think they are leading some kind of movement, but having Judy among the socked will surely help. I assume she got a full pair without having to share it with the other person in the photo.
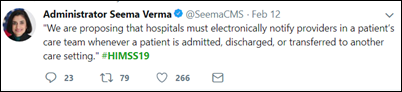
The American Hospital Association is already blasting attempts to make its members share patient data, declaring that “CMS already has better levers to ensure the exchange of appropriate health information for patients.” Hospitals will do anything to prevent attempts to make them share information — that is both infuriating and embarrassing.
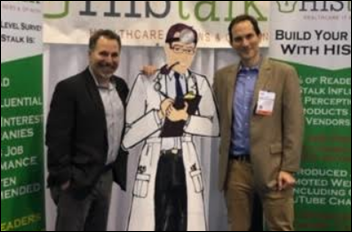
Lyle Berkowitz, MD (MDLive) and Justin Graham, MD, MS (Hearst Health) dropped by to pay their respects to their retiring colleague.
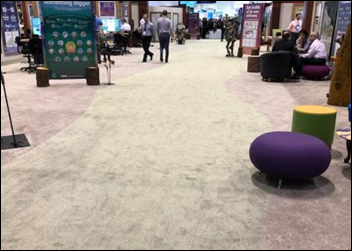
Epic’s booth was so big that they created their own aisle and demarcated it as a green, winding path. Epic booked 13,500 total square feet (behind only IBM) at a cost of around $200,000 per exhibit hall day not counting freight, furnishings, services, signage, the salary and expenses of booth staffers, and a bunch more stuff like electronics rental. Someone on the shuttle bus this morning said Epic had a large, impressive booth that must have cost a fortune, when another guy chimed in, “We’ve paid them $250 million, so we don’t care what they spend.”
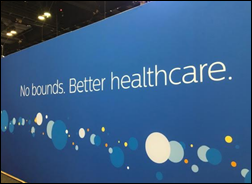
I dunno … that tall wall seems like a “bounds” to me.
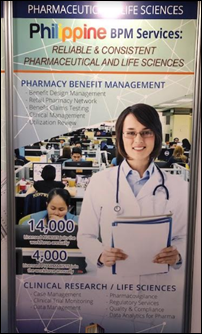
I really enjoyed talking to the super-nice and fun guys at the Philippines outsourcing booth. Vendors, they have a lot of clinicians (nurses, doctors, and even specialists and surgeons) ready to help with everything from utilization review to life sciences work, not to mention an army of well-trained folks who can supplement all kinds of projects remotely, such as revenue cycle management.
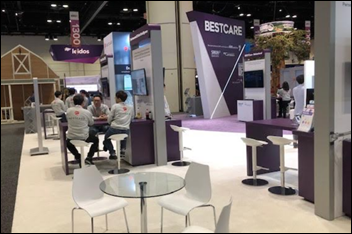
I’ve observed in past years that BestCare has a cool-looking EHR, but the obvious cultural differences between its South Korea home and the US will make it a tough sell unless it hires US salespeople. Example: I counted 15 employees and zero visitors in its large booth. A guy comes up to scarf some free BestCare popcorn, and just to be nice, asks the company’s point person, “Do you have an EHR, or is it a dashboard that sits on top of the EHR?” The BestCare rep says, “We have both” and then just looks at the guy without saying anything more for several uncomfortable seconds, after which the guy took his popcorn and ran. An American salesperson would have been projectile vomiting out a stream of product superlatives in making the visitor earn his popcorn.
I stopped by Guardian Eagle upon seeing their first-time exhibitor sign. The St. Petersburg, FL-based company offers security systems and around-the-clock monitoring.
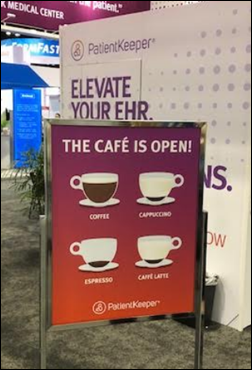
I like coffee, but I’m simple – the cheap stuff is just fine as long as there’s plenty of flavored non-dairy creamer to make it more like a milkshake (hazelnut and Almond Joy are my favorites). I know nothing about the fake Italian descriptions Starbucks assigns to its sizes and Mrs. HIStalk has to order for me since I can never remember which is Americano, espresso, cappuccino, and all those other options. I’m happy, therefore, that PatientKeeper did what every coffee shop should – skip the showy vernacular and just tell us what it means, even if Starbucks coyly makes you say something other than “large” to get them to pour your cup.
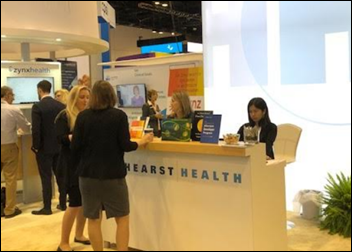
Hearst Health not only had our sign out, but the First Databank subset of the company wrote a poem for the always-popular Health Policy Valentine’s Day in honor of the last HIMSS conference for The Smokin’ Doc: “Roses are red. Violets are blue. We’ll miss you Smokin’ Doc, but we know where to find you .” I’m not saying he won’t return next year, just not in an HIStalk-paid booth. Now that I’ve killed off HIStalkapalooza and exhibiting, I’ll be asking readers next week what, if anything, we should do at HIMSS.
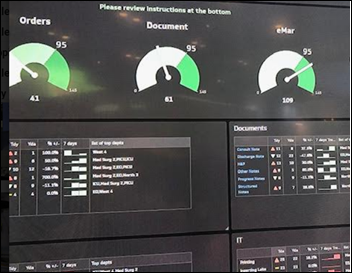
ESD is offering a new product called ARI (Virtual PM), a real-time automation tool for managing go-live activities, especially those involving contractors and consultants. The demo looked cool – consultants clock in by facial recognition, real-time updates show problem areas and workload, and trending shows which fires have been put out vs. those that need more resources. The real-time information stream helps health systems keep projects on time and budget by raising the visibility of problem areas and making sure consultants are being used optimally and billing correctly.
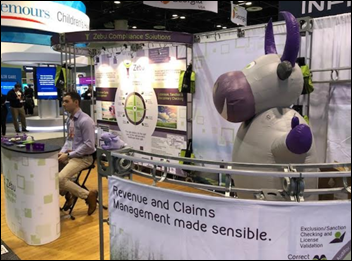
I would consider this booth to be a contender for best design and theme in a modest space.
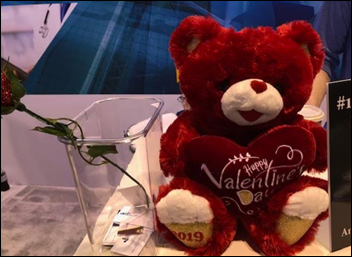
This vendor (I forget who it was) is one of few offering those little heart-shaped candies and having a drawing for this guy.
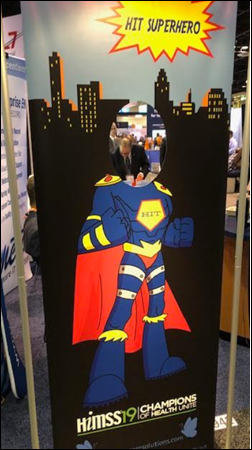
I had a creative idea for taking a picture with this cut-out, but it would have required a ladder and some privacy.
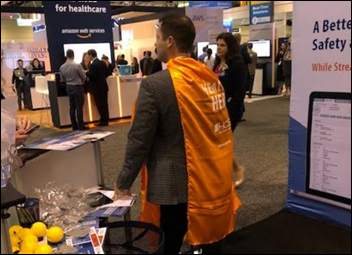
This hero didn’t explain how he earned that honor, but he must have been granted the right to assign the exalted status to others since he had a lot of giveaway capes.
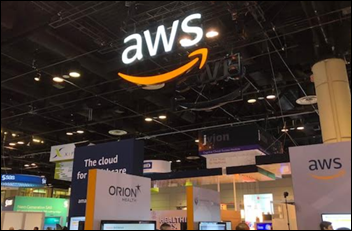
I failed to notice previously how neon-like the AWS sign is, rather like that of Seattle’s Pike Place Market.
At least eight companies were offering to take professional headshots and had the equipment and photographer to do it. Pretty cool.
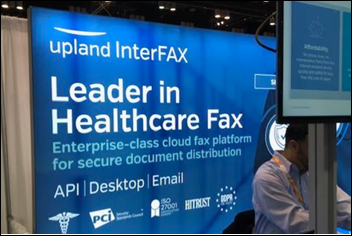
Only in healthcare would you find “enterprise-class,” “API,” and “cloud” in the same tagline as “fax.”
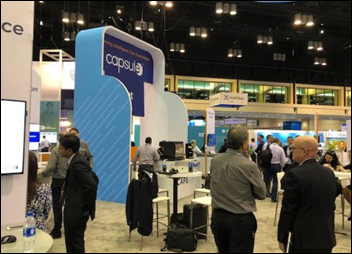
Capsule must have assumed that its freedom from Qualcomm Life shackles was inevitable since its booth looked pretty polished. The name change back to Capsule happened just a few days ago, although I don’t think it ever really went away. Although I do have one slight correction – the Francisco Partners acquisition announcement said the old name of “Capsule Technologies” would be restored, but the actual original name was the odd-looking “Capsule Technologie” because it was started in France, as I seem to recall.
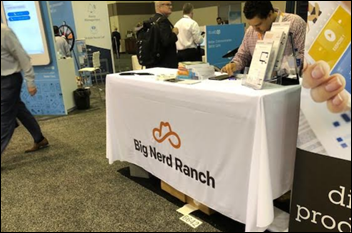
Big Nerd Ranch is my new favorite company name and logo. It offers developer training, noting that 21 of the world’s top 25 applications involve developers it trained.
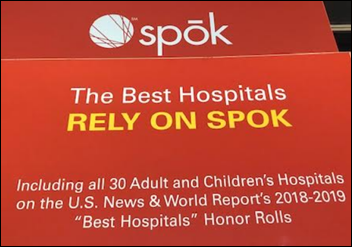
I find statements like these to be compelling.
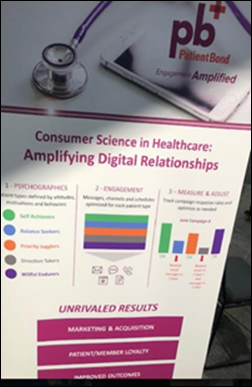
I loved talking to Brent from PatientBond. I’ve somehow lost my notes, but he told me that the company uses psychographic analysis – obtained by having patients complete a 12-question quiz — to assign them a profile and to then engage them in ways that are likely to be most effective (it’s a nudge theory kind of thing). He said that “Willful Endurers” live for the moment and will ignore messages involving long-term management of their health, but will respond to a single “do this now” message that can then queue up the next one. Most astounding is that those Willful Endurers make up two-thirds of urgent care visits (not to mention a bunch of ED visits), and private equity firms that own those centers are using the information for marketing purposes. Brent used to work for Proctor & Gamble before leaving the dark side, so he knows how products are pushed and applying that knowledge to health is cool. It’s fascinating and it works – urgent care centers are snapping it up with an average sales cycle of just 30 days (since the product is incredibly inexpensive and has a 40x ROI) but hospitals are of course dragging their feet in the usual 12-24 month decision cycles. It’s like Cambridge Analytica except for good instead of evil.
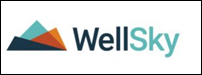
I dropped by WellSky, the new name for the former Mediware, which has assembled an impressive package of applications for home, hospital, practices, and community with 10,000 customer sites. The new CEO is Bill Miller, former CEO of OptumInsight, which became a juggernaut through well-managed acquisitions, a strong brand identity, and a relentless pursuit of growth. Not only that, I scored 10 of 10 on its trivia quiz, earning me an Amazon Echo Dot. Mediware was always kind of cool but niche-y, with no clear “what do you do exactly” identity and 30 individual brands. TPG Capital bought Mediware in December 2016 from Thoma Bravo. Annual revenue is nearly $300 million.
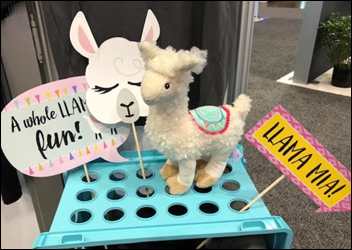
I think I just heard a collective sigh of “awwww” from some of the ladies of health IT. I can say for sure that I heard it at the Stericycle booth, where the llama tee shirts were snapped up quickly.
I sat through an interesting presentation by Verizon on location services. Scenario: a patient enters a hospital campus, at which time a welcome message and parking instructions (based on their specific campus destination) pops up on their cell phone. A virtual beacon alerts the hospital that they have arrived. They are guided to their service area with turn-by-turn, inside-the-building instructions. They have their procedure and their family is notified when they can visit. That’s amazing on its own, but even more so when you remember that just a few years ago, hospitals were adamant that cell phones had to be turned off despite a complete lack of evidence explaining why, leaving patients, visitors, and staff offline permanently while at work. The hospital cell phone ban is even more embarrassingly dark ages to me than faxing.
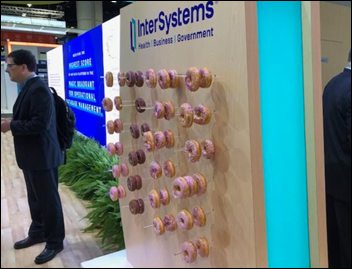
I think this is my first Wall O’ Doughnuts.
I talked to the folks at Goliath Technologies about their EHR performance monitor for Citrix and other infrastructure. It goes way beyond simple application monitoring. Here’s a real-life scenario from a hospital client. The hospital sees a massive EHR slowdown in certain areas. The app is running fine in the data center, but not on the desktop. The various IT teams – server, app, network – say everything is fine on their end (they always say that), yet the users are dying by hourglass. Some network guy takes a shot in the dark in recalling that a new driver was rolled out to every hospital scanner and suggests that each of those drivers be manually rolled back, which will take forever with the system still unavailable to users. Someone remembers that Goliath is running and checks its micro-detailed log – say, look at this, the slowdown happened early Sunday morning and seems to be network-related. Oh, Mr. Network Guy who wanted the drivers rolled back, did you make any system changes at that time? Why yes, as a matter of fact, but all we did was replace a switch that serves those specific units, although I can’t see how that would … OK, I’ll get a team on it. Goliath measures and logs everything that might degrade the Epic, Cerner, or Meditech user experience, giving IT a heads-up even before the users complain.
I checked out NextGate for an overview of the challenges and opportunities of patient matching. The state of the art is comparing current data from third-party sources to potentially stale EHR data that has outdated addresses or telephone numbers. The company offers location intelligence, where every EMPI patient address is geocoded for market research or locating the nearest doctor.
NantHealth surely has the tiniest booth ever for a publicly traded company that is also a HIMSS Diamond Member – it occupied the same 10×10 space we did.
Note to presenters: the correct answer to “do I need to use this microphone?” is always yes. Despite your self-consciousness, it’s a little bit more work for you and lot more for your audience when you insist on giving a long talk without it (even if you do preface it with a chuckling reference to how loud your voice is).
I was drawn to Indigo Vision, which offers video security cameras that can be integrated with other applications. They showed a high-resolution camera streaming real-time crowd video that could track the movement of individuals by their attributes – male, red shirt, backpack, glasses, etc. That way it’s easier to look for someone suspected after the fact of doing something by querying the metadata to find video in which a guy with a red shirt and backpack was recorded. The company’s basic video system can be programmed with rules, such as triggering an alarm if anyone enters a garage after hours.
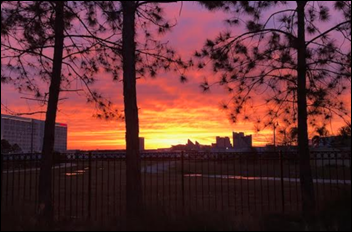
That’s a wrap on what will be the next-to-last day of HIMSS19 for most attendees who, like me, will be departing Orlando either Thursday evening or Friday morning. I’ll be back with a final day’s report tomorrow night, but if you are leaving, safe travels and Happy Valentine’s Day to you and those who are waiting patiently at home for you.
Would have liked to have seen more about Expanse here. Would like to see more about it on this site…