Top News
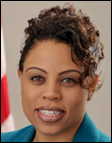
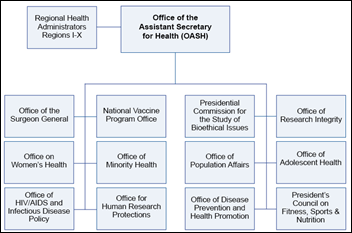
HHS Secretary Sylvia Burwell transfers National Coordinator Karen DeSalvo, MD, MPH, MSc to the position of acting assistant secretary for health — it oversees public health, including the Office of the Surgeon General — in response to the Ebola threat. DeSalvo replaces Wanda Jones. ONC COO Lisa Lewis (above) is named acting national coordinator, effective immediately. Ms. Lewis’s background is in grant management for ONC and FEMA, so her non-clinical, non-technical experience will contribute to ONC’s identity struggle in a post-Meaningful Use world. I would expect HHS to launch a search for a permanent and well-credentialed national coordinator quickly since its internal personnel stores have been recently depleted (assuming that DeSalvo’s move is permanent, which isn’t the stated case so far, which otherwise means Lewis may be keeping the seat warm for some time).
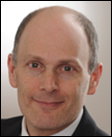
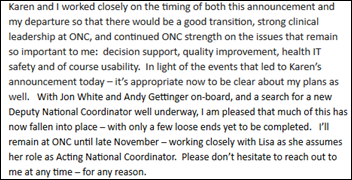
Jacob Reider, MD would seem to have been the obvious choice for interim national coordinator since he is deputy national coordinator, but he confirms with me that he has resigned, having promised to his family several weeks ago that three years of commuting to DC was enough. That leaves only Jodi Daniel and Kelly Cronin from Farzad’s 10-member team of a year ago, at least barring any additional announcements.
Reader Comments
From Frank Poggio: “Re: Karen DeSalvo reassigned from ONC. If this does not signal the end is near for the MU fed program, I do not know what would. She was there for maybe six months, came up with the grand revelation that interoperability is a bus, issued a voluminous dissertation on what was wrong, then headed for the hills! Can’t wait to see ONC /DHSS press releases on what a great job she did.” ONC was all over the Ebola issue even though the EHR turned out to be non-contributory at THR, so DeSalvo’s interest and Katrina-related public health background put her in the right place at the right time. Physicians with practice experience and an MPH from a decent school will find many job opportunities as the industry matures from encounter management to population management. I think ONC’s best purpose once they’ve either handed out all the MU money or caused providers to lose interest in receiving it would be to (a) retool EHR certification to encourage interoperability and issue standards accordingly, and (b) run with the idea of the healthcare IT patient safety center if they can get Congress to fund it. They got EHRs out in the field, now it’s time to focus on using them for patient rather than provider benefit.
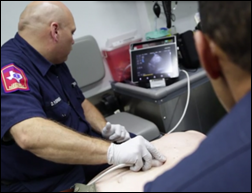
From The PACS Designer: “Re: ultrasound emergency wireless app. Samsung has demonstrated an ultrasound wireless application for emergency situations. A test showed that life saving could be achieved through the immediate sending of ultrasound images to emergency departments from ambulances.” That’s a good reminder that sometimes creating new data elements isn’t as important as moving the existing ones around more effectively to increase their value to a wider audience.
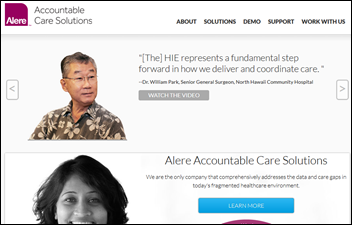
From Barry Black: “Re: Wellogic. Alere has divested the former company that was Alere Accountable Care Solutions.” Alere acquired Wellogic, founded in 1993 in late 2011. I interviewed President and CEO Sumit Nagpal a year ago. Alere ACS offers a PHR, EHR, HIE, decision support, analytics, and wellness and health coaching platforms. The company provided this response:
Based on strategic review by a leading consulting firm, Alere made a decision to refocus its energies on its main business market — diagnostics. As a part of this strategic refocus, Alere chose to divest certain assets, including those in connected health and health management. Alere ACS was the cornerstone of the Alere connected health strategy, and during its Alere tenure, enjoyed great investment that were mutually beneficial to Alere and Alere ACS — including tens of millions of dollars of enhancements to its core HIE platform. Alere ACS has now successfully separated from Alere into a new entity that will operate independently. This new entity has received a significant commitment of support and capital that will ensure continued operations and a sizable R&D investment for short- and long-term success. The new unit will continue to focus on the connected health market, including integrations with various diagnostics, mobile devices, and home monitoring opportunities. The new entity is financially robust and is armed with the necessary resources to achieve and support better healthcare and financial outcomes for the healthcare system. Executive leadership, engineering, and professional services remain unchanged.
From Lazlo Hollyfeld: “Re: non-competes. No rank-and-file employee should be subject to these agreements, and certainly not for two years.” Jimmy John’s, which is my least-favorite sub chain next to Quizno’s and not in possession of any obvious meat and bread secrets, slips a two-year non-compete clause into its employment agreement that prohibits its $8 per hour sandwich makers and delivery drivers from working not only at competing sub chains, but for any business located near one of its locations that makes 10 percent of its revenue from sandwich sales. Lawyers in a class action suit say the chain’s 2,000 locations mean that an employee who quits can’t work in an area covering 6,000 square miles. It’s like every non-compete that claims to cover non-management employees: a load of repressive corporate crap dreamed up by paranoid management that wouldn’t withstand five minutes of scrutiny in court, existing only because non-management employees don’t have the time and money to challenge it.
From Deanna: “Re: Plato’s Cave. Made me think of you and why your contribution to HIT is so much better than anyone else’s. You have been outside the cave.” The outgoing editor of The Wall Street Journal’s CIO Journal says he left journalism to work for Oracle because “journalists are at least twice removed from the essence of what they write about … I also don’t want to watch technological evolution while imprisoned in a cave, forced to take someone’s word for how it’s made and how it’s used. I want to observe it for myself.” Diligent writers often do a good job covering complicated subjects of which they have zero first-hand experience for experts who live it every day, but I get annoyed when they get lazy and just dutifully reword press releases or stray over that already generous line and start editorializing or delivering podium speeches based entirely on their cheap-seats view, like a couch potato sports fan yelling instructions to a professional football coach or a secluded porn watcher providing relationship advice.
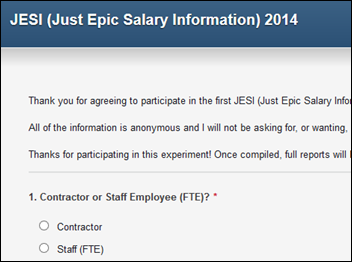
From Clinic EHR Director: “Re: Epic staff rates. Most of the information out there is overpriced, inaccurate, or both. A friend put together a survey and will publish it free. I would love it if this could be made available to as many people in the industry as possible.” The Epic salary survey is here and the results will be published here.
From P.O. Garth: “Re: HIStalkapalooza. Just curious what night it will be?” This might set the record for the earliest inquiry about an event that’s still almost six months away. HIStalkapalooza will be Monday, April 13, 2015. It will be the best HIStalkapalooza, the last, or both since I’ve decided to take the planning out of a single sponsor’s hands and instead run it myself with the help of Lorre and Jenn and the financial support of five sponsors yet to be chosen (let me know if your company is interested – you’ll get lots of exposure and invitations). Last year was the breaking point for me since ticket demand far exceeded supply and people I wasn’t able to invite got personally rude even though I spend months every year from late summer to spring sweating details for no personal benefit, leading me to swear that I was done with it. For Chicago, the facility, band, and menu are all under contract – it should be pretty great. If it’s the last one, it will at least be legendary.
HIStalk Announcements and Requests
This Week on HIStalk Practice: the DoD’s DHMSM RFP deadline is pushed back — again. Qualis Health achieves MU goal. Jerry Broderick suggests three questions to ask before joining an employed physician network. Tennessee Primary Care Association implements new pop health/analytics tools. HP interviews Rob Tennant, SVP of government affairs, MGMA. Modernizing Medicine co-founders win leadership award. Check out the HIStalk “Must-See” Exhibitors Guide for MGMA 14. Thanks for reading.
This week on HIStalk Connect: Doctors Without Borders is developing an SMS-based Ebola screening tool to engage with the local West African population. HealthTap announces that it has created a national telehealth platform that will provide virtual visits for $44 per session. XPRIZE announces 11 finalists in the Nokia Health Sensor Challenge.
Listening: new from Cold War Kids, bluesy indie rockers from Long Beach, CA.
Webinars
November 5 (Wednesday) 1:00 ET. Keeping it Clean: How Data Profiling Leads to Trusted Data. Sponsored by Encore, A Quintiles Company. Presenters: Lori Yackanicz, administrator of clinical informatics, Lehigh Valley Health Network; Randy L. Thomas, associate partner of performance analytics, Encore, A Quintiles Company; Joy Ales, MHA, BSN, RN, senior consultant, Encore, A Quintiles Company. Data dictionaries, organizational standards, and pick lists for data entry fields may describe the intent of a particular data field, but don’t guarantee that the data captured in the source system actually reflects that intent. Data profiling is the statistical analysis and assessment of the data values in source systems for consistency, uniqueness, and logic to ensure that the data landing in a data warehouse or analytic application is as expected. Attendees will learn which projects benefit from data profiling and the resources needed to accomplish it.
Acquisitions, Funding, Business, and Stock
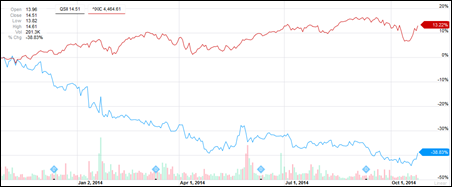
NextGen parent Quality Systems reports Q2 results: revenue up 9 percent, adjusted EPS $0.13 vs. $0.22, beating revenue expectations but missing on earnings. Above is the one-year QSII share price chart (blue, down 39 percent) vs. the Nasdaq (red, up 13 percent).
2
Cerner announces Q3 numbers: revenue up 15 percent, adjusted EPS $0.42 vs. 0.35, falling short on revenue expectations but meeting consensus earnings. Above is the one-year CERN share price chart (blue, up 6 percent) vs. the Nasdaq (red, up 13 percent). The breathy reports of $XXX billion of healthcare IT startup investment hide the fact that most of the publicly traded HIT vendors aren’t exactly killing it on Wall Street, which the irrationally exuberant cheerleaders will spin as evidence of the changing of the guard rather than the historically difficult HIT business climate.
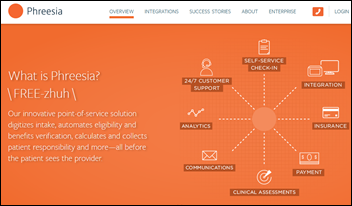
Patient self-service app vendor Phreesia raises $30 million in funding.
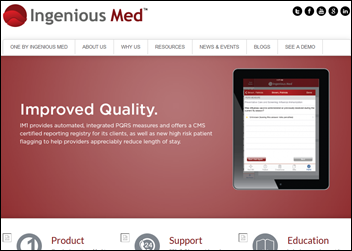
North Bridge Growth Equity becomes a majority stake owner in patient encounter platform vendor Ingenious Med with an undisclosed financial investment.
Sales
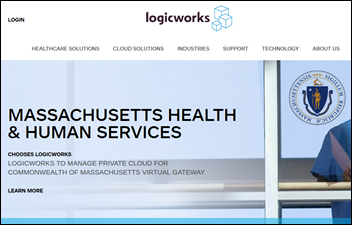
Logicworks will host the Massachusetts Health and Human Services Virtual Gateway portal.
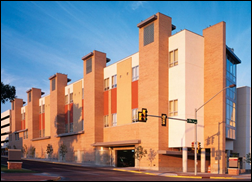
Wyoming Medical Center (WY) selects Wolters Kluwer’s ProVation MD Cardiology for structured reporting and coding in it catheterization labs.
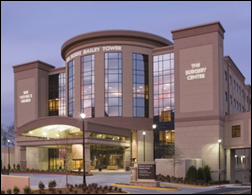
DeKalb Medical (GA) selects Connance’s Patient-Pay Optimization program to improve productivity and improve patient experience.
People
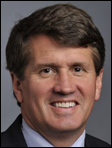
MModal names Scott MacKenzie (Experian Health) as CEO and board member.
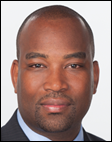
Health Data Consortium, the group that runs Health Datapalooza, names Chris Boone, PhD (Avalere Health) as executive director.
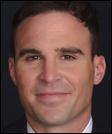
HDS hires Bradley Johnson (Caradigm) as senior executive of business development.
Announcements and Implementations
Strata Decision launches cloud-based StrataJazz Continuous Cost Improvement to help providers reduce waste and inefficiency.
Greythorn launches a healthcare IT salary survey and will donate $1 for each survey completed to Autism Speaks Foundation.
Long-term care EHR vendor HealthMEDX announces its iCare POE mobile care management system.
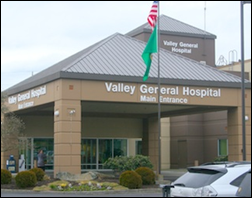
Valley General Hospital (WA) goes live on Medsphere’s OpenVista.
HSHS Medical Group (IL) launches a pilot that will test the use of Apple Watch, due out next year, in its medical home program.
Government and Politics
HHS announces the four-year, $840 million “Transforming Clinical Practice Initiative” incentive grant program to move providers to value-based, patient-centered, coordinated health services, saying that healthcare IT will be a key component. Among the suggested strategies is daily review of EHR quality and efficiency information. Specifically listed is secure, standards-based, bi-directional communication with other providers.
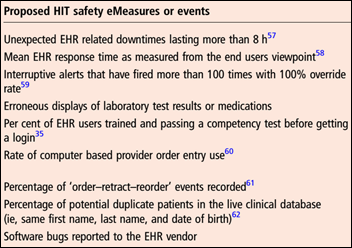
Three industry notables (Dean Sittig, David Classen, and Hardeep Singh) propose in a JAMIA article that ONC’s planned HIT Safety Center (a) create a post-marketing HIT patient safety event surveillance system; (b) develop policies and procedures for investigating those events; (c) design random safety assessments of large providers; and (d) advocate HIT safety. The surveillance function would look at system failures, inadequate design, improper user configuration or usage, interface problems, and missing or unimplemented safety-related features. I would be happy if someone would just implement an easy way (on-screen button?) for providers to communicate safety concerns directly to vendors with a CC: to a safety center. Several organizations (some of them governmental) claim to have such a system, but none get significant use because end users don’t know about them or aren’t willing to complete a pile of paperwork that doesn’t benefit them directly.
The Department of Homeland Security is reviewing possible cybersecurity flaws in medical and hospital devices (including IV pumps and cardiac devices) that could make them vulnerable to hackers, stating its intention to work with vendors to correct software problems.
Other
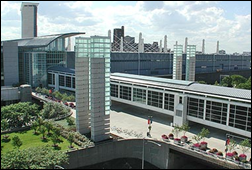
HIMSS will move its 2019 convention from Chicago to Orlando in a dispute over hotel room rates, citing its unhappiness that the RSNA conference obtained a “favored nation” clause that guarantees it the lowest room rates for events held from April through November. I surveyed readers in early 2013 about their preferred HIMSS conference cities and Chicago finished near the bottom, with only 6 percent choosing it as their favorite — New Orleans, Atlanta, Dallas, San Antonio, and Boston did as poorly or worse, while San Diego, Las Vegas, and Orlando topped the list. Chicago is easily my least-favorite convention city (even though I like visiting it otherwise) due to overpriced and indifferent hotels, surly union workers, poor public transportation to McCormick Place, and the near-certainty of cold, dreary weather in April (which of course exhibitors love since it keeps attendees inside looking at booths). HIMSS scratched its home city’s back by holding the conference there in 2009 after pushing the usual date back several weeks to avoid blizzards (which didn’t work), pulled the conference out again because of union-driven high costs of exhibiting at McCormick Place, and then ill-advisedly decided to return in 2015. Too bad their squabble comes too late to move HIMSS15 somewhere else.
Interesting: scientists nearly 10 years ago came up with an Ebola vaccine that was 100 percent effective in protecting monkeys, but the $1 billion plus cost of bringing a drug with minimal sales potential to the US market sent it to the shelf, where it remains.
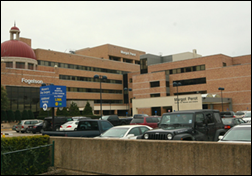
A Diagnosis article by the aforementioned Dean Sittig and Hardeep Singh, along with Divvy Upadhyay, looks at the treatment of Ebola patient Thomas Duncan at Texas Health Presbyterian Hospital Dallas, reviewing the patient’s record to find several discrepancies in THR’s announcements:
- The patient presented with a temperature spiking to 103 degrees, dizziness, GI symptoms, headache, and a self-reported pain score of eight on a 10 scale, contradicting hospital reports that his initial symptoms weren’t severe.
- The nurse documented his recent travel to Liberia.
- The ED doctor prescribed Tylenol and antibiotics (the article didn’t question why he or she prescribed antibiotics for vague symptoms that could be non-infectious or viral, but antibiotic overuse and resistance is a topic for another day).
- The authors speculate that the ED physician chose predefined phrases from EHR-suggested drop-downs that misled caregivers who read the notes later.
- They also speculate that the hospital is located next to a high-immigrant population area that a county commissioner termed “a little Ellis Island” that could have caused employees to miss the red flag of “a black man with a foreign accent who reported he came from Liberia and presented with serious ‘flu-like’ symptoms to an ED which reportedly had received CDC and county health department’s guidance as early as July 28th, 2014.”
- The article points out that clinicians often misdiagnose or miss common clinical conditions and it’s not the EHR’s job to replace their critical thinking and history-taking skills.
- It adds that doctors tend to ignore nurse-generated documentation, both on paper and in the EHR. Sad, but true.
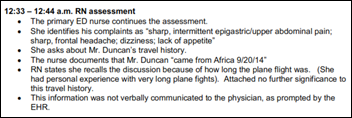
THR previously submitted to Congress a timeline of Thomas Duncan’s ED visit with some interesting items:
- Triage didn’t start until an hour after the patient’s arrival at 10:37 p.m. – he was sent to the waiting area (in contact with everybody else there) and wasn’t taken to the treatment area for for nearly 90 minutes.
- The ED physician accessed the triage nurse’s report at 12:27 a.m., but the travel history question hadn’t been asked yet since it wasn’t the triage nurse’s responsibility.
- The patient first reported his travel history to Liberia in a 12:33 a.m. question from the primary ED nurse, but she didn’t pick up on the importance of his answer and ignored the EHR prompt to verbally relay it to the ED doctor (big-time fail there).
- Audit logs show that the ED doctor reviewed the Epic sections that included the patient’s travel history several times between 12:52 and 1:10 a.m. Remember that at that point, the EHR should have been basically a single screen of information since all that had been documented was triage, the primary nurse’s initial workup, and a few vital signs. Specifically in Epic, the authors say, that includes screens for: ED lab results, Visit Navigator, related encounters, flowsheet, allergies, home meds, and ED patient history, all of which should have been pretty much blank.
- The doctor later reviewed the patient’s history in which he said he was a “local resident,” had not been in contact with sick people, and had not experienced GI symptoms (contradicting the triage nurse’s recording of his chief complaint – in other words, the patient gave incorrect and misleading information for some reason).
- The ED doctor discharged the patient with a diagnosis of sinusitis (not sure where that came from) and abdominal pain.
My conclusions: (a) Epic worked as it should have although the ED doctor still missed crucial information despite spending a lot of time looking at what should have been minimally populated Epic screens and possibly not the patient himself; (b) the hospital should have been asking travel questions at triage, which THR has since required; (c) the ED nurse missed an obvious red flag and broke hospital policy by documenting in the EHR but not reporting the travel information verbally; (d) the ED doctor either missed what should have been plainly obvious travel information or failed to note its relevance; (e) the patient told the ED doctor a very different story than he had told the nurse previously, eliminating or changing information that would have put the ED doctor on alert. All of this points out how unprepared the hospital was for detecting possible Ebola patients despite public health warnings, along with their lack of urgency to put new policies in place. My bigger conclusion: hospitals are not good at all with issues related to public health, and public health departments don’t seem to have the influence to drive sound infectious disease policy out of their ivory towers to the front lines.
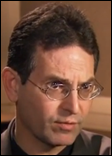
BIDMC CIO John Halamka, MD proposes that clinical documentation, which was designed for billing rather than care coordination, be redesigned around a team-based, story-oriented structure that eliminates redundancy, inaccuracy, and copied-pasted text that doesn’t convey (or even hides) the patient’s story. It resonates with me because I’m getting increasingly frustrated that EHRs are superior to paper in every way except that one – the tendency to generate a lot of worthless but structured information that masks the sometimes obvious issues, meaning those EHRs make care worse instead of better. It’s time to reclaim the EHR from administrators, bureaucrats, CMS, and malpractice attorneys and give it back to patients and clinicians. Halamka writes on his blog:
Imagine if the team at Texas Health Presbyterian jointly authored a single note each day, forcing them to read and consider all the observations made by each clinician involved in a patient’s care. There would be no cut/paste, multiple eyes would confirm the facts, and redundancy would be eliminated. As team members jointly crafted a common set of observations and a single care plan, the note would evolve into a refined consensus. There would be a single daily narrative that told the patient story. The accountable attending (there must be someone named as the team captain for treatment) would sign the jointly authored Wikipedia entry, attesting that is accurate and applying a time/date stamp for it to be added to the legal record.
After that note is authored each day, there will be key events — lab results, variation in vital signs, new patient/family care preferences, decision support alerts/reminders, and changes in condition.
Those will appear on the Facebook wall for each patient each day, showing the salient issues that occurred after the jointly authored note was signed.
With such an approach, every member of the Texas care team would have known that the patient traveled to Dallas from West Africa. Every member of the care team would understand the alerts/reminders that appeared when CDC or hospital guidelines evolved. Everyone would know the protocols for isolation and adhere to them. Of course, the patient would be a part of the Wikipedia and Facebook process, adding their own entries in real time.
A study of ICU patient alarms finds that each occupied bed generates 187 audible alarms per day, many of them false alarms related to arrhythmia. It suggests that hospitals reduce alarm fatigue by reviewing their alarm settings and consider changing some alarms from audible to text messages.
A woman who tried to kill herself by gouging out her eyes with a pencil sues LA-USC Medical Center, saying that one of its nurses took a picture of her and shared it with a friend who then posted it on a shock website.
Weird News Andy fiddles around with this story, in which a concert violinist plays his instrument on the operating room table as neurosurgeons implant a “brain pacemaker” to correct his otherwise career-ending tremors. The surgery team monitored the patient’s movements via a three-axis accelerometer as he played and they inserted electrodes into his brain to make sure they hit the right spot. It worked: three weeks later, he was back on stage with the Minnesota Orchestra.
Sponsor Updates
- Yale New Haven Health System (CT) implements SSI Group’s Audit Management solution.
- Predixion Software CEO Simon Arkell is named “Outstanding CEO” for a mid-sized company by the Orange County Technology Alliance.
- PerfectServe President and CEO Terry Edwards writes a blog post called “Prioritizing Communications to Improve Care Coordination.”
- AOD Software and Imprivata partner to provide a secure communication platform for the senior healthcare market.
- Medical Economics names ADP AdvancedMD, Allscripts, Aprima, CompuGroup Medical, e-MDs, eClinicalWorks, GE Healthcare, Greenway, Kareo, McKesson Specialty Health, NextGen, Optum, Quest Diagnostics, and RazorInsights to its “Top 50 EHRs” list.
- MedAptus will integrate Entrada’s dictation recording technology with its Pro Charge Capture solution.
- Truven Health Analytics introduces Interactive Reporting, which helps health plans analyze account-specific cost, use, and quality.
- Perceptive Software will introduce Medical Content Management at RSNA 2014.
EPtalk by Dr. Jayne
I’m always excited to receive reader mail, although I’m terribly behind on answering it. I have a couple of blogger / author friends and am convinced HIStalk has the best readers out there. That was proven this week when several of you wrote offering advice for my friend’s oncology RFP conundrum. I appreciate the input and have forwarded your thoughts.
Weird News Andy weighed in on last week’s discussion of mood-altering wearables, sharing that it “depends on who is wearing them and what else they are wearing. Mrs. Weird has an effect on my mood no matter the other variables.” I hadn’t thought of wearables in that context when I was writing last week, but that’s an important point. Despite the mass integration of technology in all facets of our lives, I still don’t understand people who wear Bluetooth headsets constantly, let alone people wandering around with Google Glass in social situations. I wonder how much we miss of the world around us because of our devices.
Reader Foie Gras wrote about this year’s Clinical Informatics board certification exam: “Thanks for your description of last year’s experience. I took the exam this past week and I want a do-over! I feel like I studied very very hard, reviewed the AMIA course, took lots of notes, and am experienced in the field, but there were definitely questions on the test with terms I did NOT know and even on some of the topics I’d studied up on. I felt they asked a very nuanced question that I just couldn’t feel comfortable with. A bit frustrating after quite the marathon and sprint of studying. Here comes the two-month wait. I really don’t want to have to study for that thing again (although yes, I learned a ton studying for it and it was really enjoyable at times.)”
I heard similar feedback from other colleagues who sat for the exam this year. Preparing for board certification can be arduous, but being able to find some enjoyment in it says something about the personality traits of those who stay in medicine. I share the frustration about some of the terminology (particularly eponyms) used on board exams. If it walks like a duck and quacks like a duck, and actually is a duck, is the fact that it’s a Baikal Teal vs. a Carolina American Wood Duck really relevant if the question is asking how many feet it has?
I enjoy leisure reading much more than I enjoy reading CMS regulations or (heaven forbid) the Federal Register, so I was excited when a colleague left a copy of “Doctored: The Disillusionment of an American Physician” on my desk. Sandeep Jauhar is a New York cardiologist. I was familiar with his first book, “Intern.” The sequel was a pretty quick read and explores several healthcare dynamics from the last two decades: the fall of fee-for-service reimbursement, providers who order diagnostic testing for their own enrichment, and fragmentation of patient care.
Although I haven’t had to deal with some of the scenarios he encountered after leaving fellowship, I’ve experienced enough of them to share some of his feelings of disillusionment. In addition to being about the “mid-life crisis” facing medicine since the creation of Medicare in the 1960s, it also covers his own mid-life crisis, which makes some sections a little difficult to read. Still, I appreciate his candor and his willingness to stick his neck out as he shares his story.
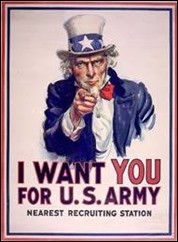
I’m used to getting a variety of mailers and postcards from vendors and recruiters, particularly around HIMSS and other conferences. I was surprised this week to get a recruiting postcard from Uncle Sam. I’m sure the mailing was set up weeks ago, but the statement “because of the wide scope of the Army’s activities, you may have the chance to see and study diseases that are not usually encountered in civilian practice” to be very timely. Some of my best friends are currently or have been military physicians. I am grateful for their service and for the sacrifice of everyone serving in all branches of the military. Veterans Day is approaching, so make plans to thank your colleagues, neighbors, and family members who have served.
Contacts
Mr. H, Lorre, Jennifer, Dr. Jayne, Dr. Gregg, Lt. Dan, Dr. Travis.
More news: HIStalk Practice, HIStalk Connect.
Get HIStalk updates.
Contact us online.
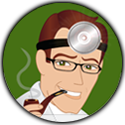
Honest question: are there EHRs left that DON'T already have a "sex assigned at birth" field? Is the practical effect…